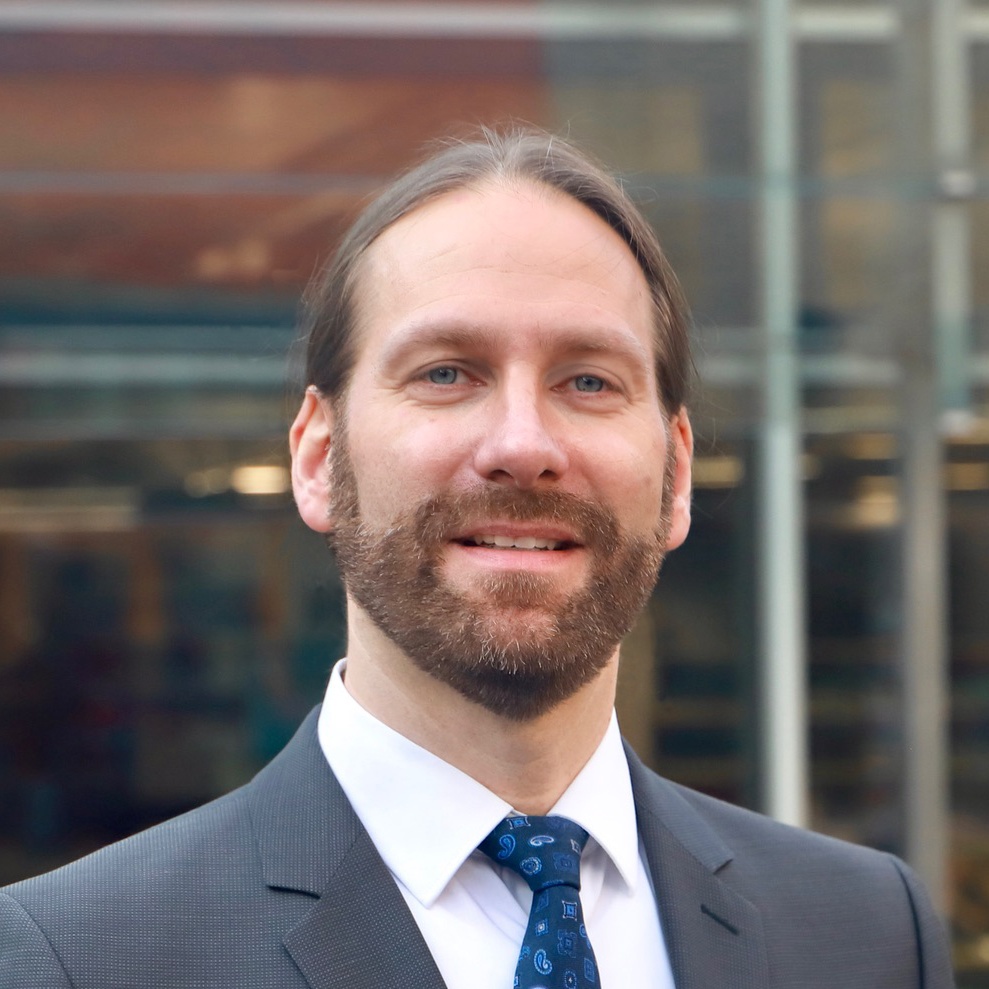
Prof. Dr. Stephan Scheele
Vision
The overarching vision is the development of robust, efficient and comprehensible methods of artificial intelligence and their transfer to industrial applications.
Explainable AI and neurosymbolic methods
The aim of this research area is to develop methods that combine machine learning with knowledge-based techniques to make AI decision-making processes more comprehensible and transparent. In particular, this includes methods of Explanatory Interactive Machine Learning (AAIML), in which explainable AI (XAI) is combined with interactive, neurosymbolic approaches. In this way, human experts can interpret model decisions, actively adapt them and improve them through continuous feedback.
A central aspect is the semantic representation of background knowledge: By using ontologies, knowledge graphs and logic-based calculations, data is not only structured but also enriched with logical information. Constructive modal logics also enable precise modelling of dynamic and uncertain knowledge, which is particularly relevant for applications with variable framework conditions (e.g. in adaptive production processes, dynamic supply chains or medical systems).
Current research addresses, for example, the use of these methods in process mining to identify hidden structures, optimisation and automation potentials and to enable informed decision-making.
The interaction of large language models (LLMs) with background knowledge and interactive machine learning is another approach that is currently being researched and used in areas such as auditing. The aim of generative data storytelling is to use extensive data sets and domain knowledge to create coherent, informative and appealing narratives that appeal to both experts and users, thus making complex data analyses broadly accessible and understandable.
Energy-efficient machine learning
Another area of research focuses on the more efficient and sustainable design of machine learning to make complex AI systems usable on embedded platforms and mobile devices. The aim is to develop algorithms that offer high performance despite limited computing resources and energy availability.
The research findings are directly applied in sensor technology, condition monitoring, monitoring systems, measurement technology and medical diagnostic systems.
Fields of interest
Modal logic and descriptive logic
Deductive systems
Explainable AI
Big Data
Knowledge representation
Human-Machine-Interaction
Software technology and architecture
Embedded systems
Edge AI
Safety-critical interactive systems
Synchronous and functional programming
Contact
+49 (0) 941 943-70243
stephan.scheele@oth-regensburg.de